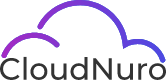
Book a Demo
Thank you! Your submission has been received!
Oops! Something went wrong while submitting the form.
Introduction
Generative AI (GenAI) tools have rapidly transformed enterprises’ operations, from automated content creation and customer service to internal productivity enhancements. Tools like OpenAI’s ChatGPT, Anthropic’s Claude, Google’s Gemini, and GitHub Copilot have become staples in enterprise workflows across industries. But with great power comes great responsibility, and risk.
While these technologies unlock new levels of automation and creativity, they also introduce unique challenges. Enterprises now face new risks such as hallucinated outputs, biased responses, prompt injection attacks, intellectual property leakage, and unauthorized API usage. In addition, the tightening regulations under frameworks like the EU AI Act, ISO 42001, and the NIST AI Risk Management Framework (AI RMF) have made it clear that governing GenAI usage is no longer optional.
That’s where generative AI governance tools come in.
These platforms help enterprises control how AI is used across departments, ensuring that outputs are ethical, secure, and compliant. From prompt monitoring and output filtering to access controls, audit readiness, and regulatory alignment, the right tools make it possible to use GenAI responsibly, at scale.
This 2025 guide explores the top 10 generative AI governance tools to help your enterprise manage risk, support compliance, and drive trustworthy AI adoption. Whether you’re overseeing data science, security, compliance, or enterprise applications, these tools protect your business and your users.
What Is Generative AI Governance?
Generative AI governance refers to the systems, policies, tools, and practices designed to ensure that generative AI technologies are used ethically, securely, and in alignment with organizational and regulatory requirements. It is a critical layer of oversight as enterprises integrate GenAI into business operations, customer engagement, and decision-making processes.
Unlike traditional AI governance, which focuses on static models trained on structured data, GenAI governance deals with dynamic, real-time interactions where outputs can change depending on user prompts, temperature settings, and API context. It introduces unique risks, such as:
To address these risks, GenAI governance encompasses several core capabilities:
Key Components of Generative AI Governance:
GenAI Governance vs. Traditional AI Governance
As GenAI models become more widely embedded into SaaS tools, developer workflows, and customer-facing experiences, governing their usage becomes essential to mitigate risk and build trust.
Must-Have Features in Generative AI Governance Tools
With enterprises integrating Generative AI into productivity suites, developer platforms, and customer-facing apps, choosing the right governance solution is critical. Effective governance ensures secure generative AI use while supporting compliance, transparency, and trust, whether using ChatGPT, Google Gemini, Claude, or open-source models like LLaMA or Mistral.
Here are the must-have features to look for in enterprise-ready generative AI governance tools:
1. Multi-Model Support (OpenAI, Claude, Gemini, Mistral, LLaMA)
Enterprises rarely rely on a single AI model. Tools must support multi-model governance across public APIs (e.g., OpenAI, Anthropic), proprietary deployments (e.g., Azure OpenAI), and open-source LLMs (e.g., Mistral, Falcon). It ensures centralized policy enforcement regardless of which model is used.
2. Prompt Inspection & Response Logging
Every prompt and its corresponding output should be captured, tagged, and stored, enabling replayability, usage tracking, and audit trail generation. It is essential for:
Some tools also classify prompts based on risk level (e.g., HR-related, legal, confidential).
3. Output Moderation (Bias, Toxicity, Hallucination Detection)
Real-time moderation engines score each output for:
It allows for the blocking or flagging unsafe responses before they reach end users, especially vital in finance, healthcare, and public sector use cases.
4. Granular Access Control & Usage Restrictions
Implement role-based access control (RBAC) to manage:
For example, only the legal team may access a legal assistant model, while the marketing team is restricted to summarization tools.
5. Integration with IAM, DLP & SSO
To ensure consistency with existing enterprise security, governance tools should integrate with:
It helps unify SaaS-based GenAI usage within your broader security architecture.
6. Compliance-Driven Policy Templates
Top platforms come with prebuilt policy templates for:
These templates include logging requirements, model transparency criteria, risk thresholds, and usage boundaries for regulated domains.
7. Risk Scoring & Approval Workflows
Each GenAI request (especially from LLMs exposed via internal portals or APIs) can be risk-scored based on:
Tools offer approval workflows for high-risk prompts (e.g., legal or customer-facing content), enabling human oversight before deployment.
Top 10 Generative AI Governance Tools
The following ten platforms are leading the charge in generative AI governance for 2025. Each offers a unique mix of compliance, security, and oversight capabilities, suitable for different organizational needs and maturity levels.
1. Lakera
Overview:
Lakera offers real-time protection for generative AI applications through prompt inspection, injection attack prevention, and output risk filtering. Its flagship tool, Lakera Guard, is a plug-and-play GenAI firewall tailored for developers embedding LLMs into enterprise apps.
Key Governance Features:
Strengths:
Limitations:
Best For:
Product security teams, LLM app developers, and SaaS vendors
G2 Rating: 5/5 (1 review)
Gartner Rating: Not yet listed
Screenshot:
2. Credo AI
Overview:
Credo AI provides a comprehensive AI governance platform for responsible AI development and deployment. It centralizes your organization's risk assessments, compliance scorecards, and policy workflows.
Key Governance Features:
Strengths:
Limitations:
Best For:
Risk managers, compliance officers, and AI ethics teams in large enterprises
G2 Rating: 5/5 (1 review)
Screenshot:
3. CalypsoAI
Overview:
CalypsoAI offers a GenAI security gateway focused on securing prompts and enforcing AI usage policies in highly regulated environments. Known for its Pentagon partnerships, it emphasizes risk-based access to LLMs and audit readiness.
Key Governance Features:
Strengths:
Limitations:
Best For:
Defense, aerospace, financial services, and high-risk enterprise environments
G2 Rating: 3.5/5 (1 review)
Gartner Rating: 4.0/5 (1 review)
Screenshot:
4. Arthur
Overview:
Arthur focuses on LLM observability and responsible model performance across production pipelines. It provides deep insights into bias, drift, hallucination frequency, and fairness across generative models.
Key Governance Features:
Strengths:
Limitations:
Best For:
Data science leads, ML Ops teams, and internal LLM platform owners
G2 Rating: 4.8/5 (19 reviews)
Screenshot:
5. Aporia
Overview:
Aporia enables real-time detection of hallucinations, bias, and data drift in GenAI systems. It’s popular among machine learning engineers building custom applications with open-source or proprietary models.
Key Governance Features:
Strengths:
Limitations:
Best For:
ML engineers and data scientists managing live LLM deployments
G2 Rating: 4.8/5 (61 reviews)
Gartner Rating: 3.6/5 (8 reviews)
Screenshot:
6. Holistic AI
Overview:
Holistic AI delivers an enterprise-grade governance framework aligned with the EU AI Act. It provides risk assessment models, explainability engines, and customizable governance workflows to ensure ethical and compliant AI operations.
Key Governance Features:
Strengths:
Limitations:
Best For:
Legal, ethics, and compliance teams with large model inventories
G2 Rating: 5/5 (3 reviews)
Screenshot:
7. Fiddler AI
Overview:
Fiddler AI is a pioneer in explainable AI (XAI) and model transparency. Its platform empowers organizations to build trust in their GenAI outputs by offering explainability, fairness analysis, and regulatory-aligned governance tools.
Key Governance Features:
Strengths:
Limitations:
Best For:
Enterprises requiring transparency in high-stakes decision-making (e.g., loan approvals, diagnostics)
G2 Rating: 4.3/5 (2 reviews)
Screenshot:
8. Monitaur
Overview:
Monitaur is an AI governance and assurance platform purpose-built for documenting, validating, and controlling the behavior of AI models, especially in heavily regulated sectors.
Key Governance Features:
Strengths:
Limitations:
Best For:
Financial services, insurance, and healthcare organizations need audit assurance.
Screenshot:
9. Zion.AI (Emerging Player)
Overview:
Zion.AI is a rising player offering lightweight, real-time LLM behavior monitoring for smaller teams and early-stage companies. It excels in prompt activity tracking, API access gating, and alerting for anomalous usage.
Key Governance Features:
Strengths:
Limitations:
Best For:
R&D labs, AI startups, and SMBs with LLM experimentation needs
G2 Rating: 4.8/5 (6 reviews)
Screenshot:
10. Mozart Data – AI Risk Governance Module
Overview:
Mozart Data, known for data pipeline automation, now includes a dedicated AI Risk Governance module to oversee data flow into generative AI systems and ensure safe, auditable outputs.
Key Governance Features:
Strengths:
Limitations:
Best For:
Data engineering teams and privacy officers are managing LLM training and input pipelines.
G2 Rating: 4.6/5 (68 reviews)
Gartner Rating: 4.9/5 (24 reviews)
Screenshot:
Comparison Table: GenAI Governance Tools at a Glance
Best Practices for Enterprise GenAI Governance (~400–500 words)
Deploying generative AI at scale requires more than selecting the right tools; it demands a mature governance strategy integrating people, processes, and platforms. The following best practices can help CISOs, AI Governance Leads, and ML Ops teams securely and ethically manage GenAI usage across departments and cloud environments.
1. Establish Cross-Functional AI Governance Committees
AI governance is not solely a technical responsibility. Form a multi-disciplinary governance team that includes:
It ensures policy decisions reflect ethical, legal, and operational perspectives and that adoption is aligned with enterprise values and regulatory expectations.
2. Implement Role-Based Access to GenAI Models
Not all teams need access to every AI tool. Use RBAC (Role-Based Access Control) to define:
Pairing model access with existing SSO and IAM platforms ensures that only authorized users can initiate GenAI queries and prevents shadow usage.
3. Monitor Prompts and Outputs with Traceability
Governance doesn’t end at access, and it extends to usage. Best-in-class tools offer prompt-to-output traceability, enabling organizations to:
Use this visibility to inform training, refine prompt templates, and improve usage controls.
4. Align Tools to Regulatory Frameworks
Adopt governance tools that map directly to key frameworks, such as:
It supports compliance and accelerates procurement, audit readiness, and vendor assessments.
5. Automate Risk Scoring and Human Approval Workflows
Some GenAI use cases, like generating legal opinions or customer-facing responses, warrant extra scrutiny. Use tools with automated risk scoring and approval workflows to:
6. Use CloudNuro.ai to Monitor SaaS-Based GenAI Tools
Even if you’ve secured your internal GenAI models, risks still emerge from SaaS integrations like:
CloudNuro.ai provides centralized visibility into GenAI usage across SaaS environments, helping security teams detect shadow tools, automate license governance, and enforce usage boundaries.
FAQs: Generative AI Governance Explained
Q1: Do we need a governance tool if we only use Microsoft Copilot or ChatGPT?
Yes. While these platforms provide basic admin controls, they don’t offer deep enterprise-grade governance features like prompt logging, risk scoring, or policy enforcement. Without third-party governance tools, you lack visibility into:
For regulated industries, relying solely on native tools leaves significant blind spots.
Q2: How do governance platforms detect hallucinations, bias, or toxic output?
Most governance tools integrate output monitoring engines that analyze:
These tools flag or block high-risk responses in real time, especially valuable in customer-facing or compliance-sensitive environments.
Q3: Can these tools work across different models like OpenAI, Claude, and Gemini?
Yes, most support multi-model governance. The best tools provide connectors or gateways that standardize governance across:
It ensures consistent policy enforcement regardless of your team's model or vendor.
Q4: How do these platforms support compliance with regulations like the EU AI Act or ISO 42001?
Top governance platforms embed policy templates and controls aligned to regulatory frameworks, including:
They help generate documentation that supports external audits and internal controls, critical for compliance with the EU AI Act, NIST AI RMF, ISO/IEC 42001, and GDPR.
Q5: What’s the difference between AI observability and AI governance?
Ideally, your platform should do both, but governance is the foundation for secure and responsible AI adoption.
Why CloudNuro.ai Complements GenAI Governance Tools
While GenAI governance platforms monitor prompt behavior, output risks, and model accountability, most lack visibility into where and how generative AI is used across SaaS ecosystems.
That’s where CloudNuro.ai steps in.
CloudNuro.ai is a SaaS governance and visibility platform that helps enterprises discover, monitor, and manage generative AI usage within business tools like:
While LLM firewalls and policy engines focus on prompt-level governance, CloudNuro.ai ensures broader AI access control and license accountability across departments.
Key Capabilities That Complement GenAI Governance:
Whether you're trying to identify unauthorized AI usage, optimize licensing, or close the loop between security and finance, CloudNuro.ai connects the dots between SaaS sprawl and GenAI risk.
Together with your GenAI governance tools, CloudNuro.ai forms a comprehensive layer of defense:
Conclusion
Generative AI has moved from experimentation to enterprise adoption fast. However, as organizations race to integrate tools like ChatGPT, Copilot, Claude, and Gemini, the need for ethical, explainable, and compliant AI governance has never been greater.
Without governance, generative AI becomes a liability:
The good news? You now have a maturing ecosystem of Generative AI governance tools that bring visibility, accountability, and control into your AI strategy. Solutions like Lakera, Credo AI, CalypsoAI, and Fiddler are leading the way from real-time prompt firewalls and output monitoring to bias detection and audit readiness.
But that’s just one side of the coin.
To truly secure your enterprise AI footprint, you must also govern how AI is used across SaaS environments, from Microsoft 365 Copilot to ChatGPT Pro. That’s where CloudNuro.ai comes in.
Ready to Secure and Govern Your GenAI Stack?
CloudNuro.ai helps you track GenAI usage across your entire SaaS ecosystem, ensuring license control, user accountability, and cost optimization.
➡️ Book a Free Demo to discover your GenAI footprint and start governing it today.
Request a no cost, no obligation free assessment —just 15 minutes to savings!
Get StartedIntroduction
Generative AI (GenAI) tools have rapidly transformed enterprises’ operations, from automated content creation and customer service to internal productivity enhancements. Tools like OpenAI’s ChatGPT, Anthropic’s Claude, Google’s Gemini, and GitHub Copilot have become staples in enterprise workflows across industries. But with great power comes great responsibility, and risk.
While these technologies unlock new levels of automation and creativity, they also introduce unique challenges. Enterprises now face new risks such as hallucinated outputs, biased responses, prompt injection attacks, intellectual property leakage, and unauthorized API usage. In addition, the tightening regulations under frameworks like the EU AI Act, ISO 42001, and the NIST AI Risk Management Framework (AI RMF) have made it clear that governing GenAI usage is no longer optional.
That’s where generative AI governance tools come in.
These platforms help enterprises control how AI is used across departments, ensuring that outputs are ethical, secure, and compliant. From prompt monitoring and output filtering to access controls, audit readiness, and regulatory alignment, the right tools make it possible to use GenAI responsibly, at scale.
This 2025 guide explores the top 10 generative AI governance tools to help your enterprise manage risk, support compliance, and drive trustworthy AI adoption. Whether you’re overseeing data science, security, compliance, or enterprise applications, these tools protect your business and your users.
What Is Generative AI Governance?
Generative AI governance refers to the systems, policies, tools, and practices designed to ensure that generative AI technologies are used ethically, securely, and in alignment with organizational and regulatory requirements. It is a critical layer of oversight as enterprises integrate GenAI into business operations, customer engagement, and decision-making processes.
Unlike traditional AI governance, which focuses on static models trained on structured data, GenAI governance deals with dynamic, real-time interactions where outputs can change depending on user prompts, temperature settings, and API context. It introduces unique risks, such as:
To address these risks, GenAI governance encompasses several core capabilities:
Key Components of Generative AI Governance:
GenAI Governance vs. Traditional AI Governance
As GenAI models become more widely embedded into SaaS tools, developer workflows, and customer-facing experiences, governing their usage becomes essential to mitigate risk and build trust.
Must-Have Features in Generative AI Governance Tools
With enterprises integrating Generative AI into productivity suites, developer platforms, and customer-facing apps, choosing the right governance solution is critical. Effective governance ensures secure generative AI use while supporting compliance, transparency, and trust, whether using ChatGPT, Google Gemini, Claude, or open-source models like LLaMA or Mistral.
Here are the must-have features to look for in enterprise-ready generative AI governance tools:
1. Multi-Model Support (OpenAI, Claude, Gemini, Mistral, LLaMA)
Enterprises rarely rely on a single AI model. Tools must support multi-model governance across public APIs (e.g., OpenAI, Anthropic), proprietary deployments (e.g., Azure OpenAI), and open-source LLMs (e.g., Mistral, Falcon). It ensures centralized policy enforcement regardless of which model is used.
2. Prompt Inspection & Response Logging
Every prompt and its corresponding output should be captured, tagged, and stored, enabling replayability, usage tracking, and audit trail generation. It is essential for:
Some tools also classify prompts based on risk level (e.g., HR-related, legal, confidential).
3. Output Moderation (Bias, Toxicity, Hallucination Detection)
Real-time moderation engines score each output for:
It allows for the blocking or flagging unsafe responses before they reach end users, especially vital in finance, healthcare, and public sector use cases.
4. Granular Access Control & Usage Restrictions
Implement role-based access control (RBAC) to manage:
For example, only the legal team may access a legal assistant model, while the marketing team is restricted to summarization tools.
5. Integration with IAM, DLP & SSO
To ensure consistency with existing enterprise security, governance tools should integrate with:
It helps unify SaaS-based GenAI usage within your broader security architecture.
6. Compliance-Driven Policy Templates
Top platforms come with prebuilt policy templates for:
These templates include logging requirements, model transparency criteria, risk thresholds, and usage boundaries for regulated domains.
7. Risk Scoring & Approval Workflows
Each GenAI request (especially from LLMs exposed via internal portals or APIs) can be risk-scored based on:
Tools offer approval workflows for high-risk prompts (e.g., legal or customer-facing content), enabling human oversight before deployment.
Top 10 Generative AI Governance Tools
The following ten platforms are leading the charge in generative AI governance for 2025. Each offers a unique mix of compliance, security, and oversight capabilities, suitable for different organizational needs and maturity levels.
1. Lakera
Overview:
Lakera offers real-time protection for generative AI applications through prompt inspection, injection attack prevention, and output risk filtering. Its flagship tool, Lakera Guard, is a plug-and-play GenAI firewall tailored for developers embedding LLMs into enterprise apps.
Key Governance Features:
Strengths:
Limitations:
Best For:
Product security teams, LLM app developers, and SaaS vendors
G2 Rating: 5/5 (1 review)
Gartner Rating: Not yet listed
Screenshot:
2. Credo AI
Overview:
Credo AI provides a comprehensive AI governance platform for responsible AI development and deployment. It centralizes your organization's risk assessments, compliance scorecards, and policy workflows.
Key Governance Features:
Strengths:
Limitations:
Best For:
Risk managers, compliance officers, and AI ethics teams in large enterprises
G2 Rating: 5/5 (1 review)
Screenshot:
3. CalypsoAI
Overview:
CalypsoAI offers a GenAI security gateway focused on securing prompts and enforcing AI usage policies in highly regulated environments. Known for its Pentagon partnerships, it emphasizes risk-based access to LLMs and audit readiness.
Key Governance Features:
Strengths:
Limitations:
Best For:
Defense, aerospace, financial services, and high-risk enterprise environments
G2 Rating: 3.5/5 (1 review)
Gartner Rating: 4.0/5 (1 review)
Screenshot:
4. Arthur
Overview:
Arthur focuses on LLM observability and responsible model performance across production pipelines. It provides deep insights into bias, drift, hallucination frequency, and fairness across generative models.
Key Governance Features:
Strengths:
Limitations:
Best For:
Data science leads, ML Ops teams, and internal LLM platform owners
G2 Rating: 4.8/5 (19 reviews)
Screenshot:
5. Aporia
Overview:
Aporia enables real-time detection of hallucinations, bias, and data drift in GenAI systems. It’s popular among machine learning engineers building custom applications with open-source or proprietary models.
Key Governance Features:
Strengths:
Limitations:
Best For:
ML engineers and data scientists managing live LLM deployments
G2 Rating: 4.8/5 (61 reviews)
Gartner Rating: 3.6/5 (8 reviews)
Screenshot:
6. Holistic AI
Overview:
Holistic AI delivers an enterprise-grade governance framework aligned with the EU AI Act. It provides risk assessment models, explainability engines, and customizable governance workflows to ensure ethical and compliant AI operations.
Key Governance Features:
Strengths:
Limitations:
Best For:
Legal, ethics, and compliance teams with large model inventories
G2 Rating: 5/5 (3 reviews)
Screenshot:
7. Fiddler AI
Overview:
Fiddler AI is a pioneer in explainable AI (XAI) and model transparency. Its platform empowers organizations to build trust in their GenAI outputs by offering explainability, fairness analysis, and regulatory-aligned governance tools.
Key Governance Features:
Strengths:
Limitations:
Best For:
Enterprises requiring transparency in high-stakes decision-making (e.g., loan approvals, diagnostics)
G2 Rating: 4.3/5 (2 reviews)
Screenshot:
8. Monitaur
Overview:
Monitaur is an AI governance and assurance platform purpose-built for documenting, validating, and controlling the behavior of AI models, especially in heavily regulated sectors.
Key Governance Features:
Strengths:
Limitations:
Best For:
Financial services, insurance, and healthcare organizations need audit assurance.
Screenshot:
9. Zion.AI (Emerging Player)
Overview:
Zion.AI is a rising player offering lightweight, real-time LLM behavior monitoring for smaller teams and early-stage companies. It excels in prompt activity tracking, API access gating, and alerting for anomalous usage.
Key Governance Features:
Strengths:
Limitations:
Best For:
R&D labs, AI startups, and SMBs with LLM experimentation needs
G2 Rating: 4.8/5 (6 reviews)
Screenshot:
10. Mozart Data – AI Risk Governance Module
Overview:
Mozart Data, known for data pipeline automation, now includes a dedicated AI Risk Governance module to oversee data flow into generative AI systems and ensure safe, auditable outputs.
Key Governance Features:
Strengths:
Limitations:
Best For:
Data engineering teams and privacy officers are managing LLM training and input pipelines.
G2 Rating: 4.6/5 (68 reviews)
Gartner Rating: 4.9/5 (24 reviews)
Screenshot:
Comparison Table: GenAI Governance Tools at a Glance
Best Practices for Enterprise GenAI Governance (~400–500 words)
Deploying generative AI at scale requires more than selecting the right tools; it demands a mature governance strategy integrating people, processes, and platforms. The following best practices can help CISOs, AI Governance Leads, and ML Ops teams securely and ethically manage GenAI usage across departments and cloud environments.
1. Establish Cross-Functional AI Governance Committees
AI governance is not solely a technical responsibility. Form a multi-disciplinary governance team that includes:
It ensures policy decisions reflect ethical, legal, and operational perspectives and that adoption is aligned with enterprise values and regulatory expectations.
2. Implement Role-Based Access to GenAI Models
Not all teams need access to every AI tool. Use RBAC (Role-Based Access Control) to define:
Pairing model access with existing SSO and IAM platforms ensures that only authorized users can initiate GenAI queries and prevents shadow usage.
3. Monitor Prompts and Outputs with Traceability
Governance doesn’t end at access, and it extends to usage. Best-in-class tools offer prompt-to-output traceability, enabling organizations to:
Use this visibility to inform training, refine prompt templates, and improve usage controls.
4. Align Tools to Regulatory Frameworks
Adopt governance tools that map directly to key frameworks, such as:
It supports compliance and accelerates procurement, audit readiness, and vendor assessments.
5. Automate Risk Scoring and Human Approval Workflows
Some GenAI use cases, like generating legal opinions or customer-facing responses, warrant extra scrutiny. Use tools with automated risk scoring and approval workflows to:
6. Use CloudNuro.ai to Monitor SaaS-Based GenAI Tools
Even if you’ve secured your internal GenAI models, risks still emerge from SaaS integrations like:
CloudNuro.ai provides centralized visibility into GenAI usage across SaaS environments, helping security teams detect shadow tools, automate license governance, and enforce usage boundaries.
FAQs: Generative AI Governance Explained
Q1: Do we need a governance tool if we only use Microsoft Copilot or ChatGPT?
Yes. While these platforms provide basic admin controls, they don’t offer deep enterprise-grade governance features like prompt logging, risk scoring, or policy enforcement. Without third-party governance tools, you lack visibility into:
For regulated industries, relying solely on native tools leaves significant blind spots.
Q2: How do governance platforms detect hallucinations, bias, or toxic output?
Most governance tools integrate output monitoring engines that analyze:
These tools flag or block high-risk responses in real time, especially valuable in customer-facing or compliance-sensitive environments.
Q3: Can these tools work across different models like OpenAI, Claude, and Gemini?
Yes, most support multi-model governance. The best tools provide connectors or gateways that standardize governance across:
It ensures consistent policy enforcement regardless of your team's model or vendor.
Q4: How do these platforms support compliance with regulations like the EU AI Act or ISO 42001?
Top governance platforms embed policy templates and controls aligned to regulatory frameworks, including:
They help generate documentation that supports external audits and internal controls, critical for compliance with the EU AI Act, NIST AI RMF, ISO/IEC 42001, and GDPR.
Q5: What’s the difference between AI observability and AI governance?
Ideally, your platform should do both, but governance is the foundation for secure and responsible AI adoption.
Why CloudNuro.ai Complements GenAI Governance Tools
While GenAI governance platforms monitor prompt behavior, output risks, and model accountability, most lack visibility into where and how generative AI is used across SaaS ecosystems.
That’s where CloudNuro.ai steps in.
CloudNuro.ai is a SaaS governance and visibility platform that helps enterprises discover, monitor, and manage generative AI usage within business tools like:
While LLM firewalls and policy engines focus on prompt-level governance, CloudNuro.ai ensures broader AI access control and license accountability across departments.
Key Capabilities That Complement GenAI Governance:
Whether you're trying to identify unauthorized AI usage, optimize licensing, or close the loop between security and finance, CloudNuro.ai connects the dots between SaaS sprawl and GenAI risk.
Together with your GenAI governance tools, CloudNuro.ai forms a comprehensive layer of defense:
Conclusion
Generative AI has moved from experimentation to enterprise adoption fast. However, as organizations race to integrate tools like ChatGPT, Copilot, Claude, and Gemini, the need for ethical, explainable, and compliant AI governance has never been greater.
Without governance, generative AI becomes a liability:
The good news? You now have a maturing ecosystem of Generative AI governance tools that bring visibility, accountability, and control into your AI strategy. Solutions like Lakera, Credo AI, CalypsoAI, and Fiddler are leading the way from real-time prompt firewalls and output monitoring to bias detection and audit readiness.
But that’s just one side of the coin.
To truly secure your enterprise AI footprint, you must also govern how AI is used across SaaS environments, from Microsoft 365 Copilot to ChatGPT Pro. That’s where CloudNuro.ai comes in.
Ready to Secure and Govern Your GenAI Stack?
CloudNuro.ai helps you track GenAI usage across your entire SaaS ecosystem, ensuring license control, user accountability, and cost optimization.
➡️ Book a Free Demo to discover your GenAI footprint and start governing it today.
Request a no cost, no obligation free assessment —just 15 minutes to savings!
Get StartedRecognized Leader in SaaS Management Platforms by Info-Tech SoftwareReviews